Signals in the Noise
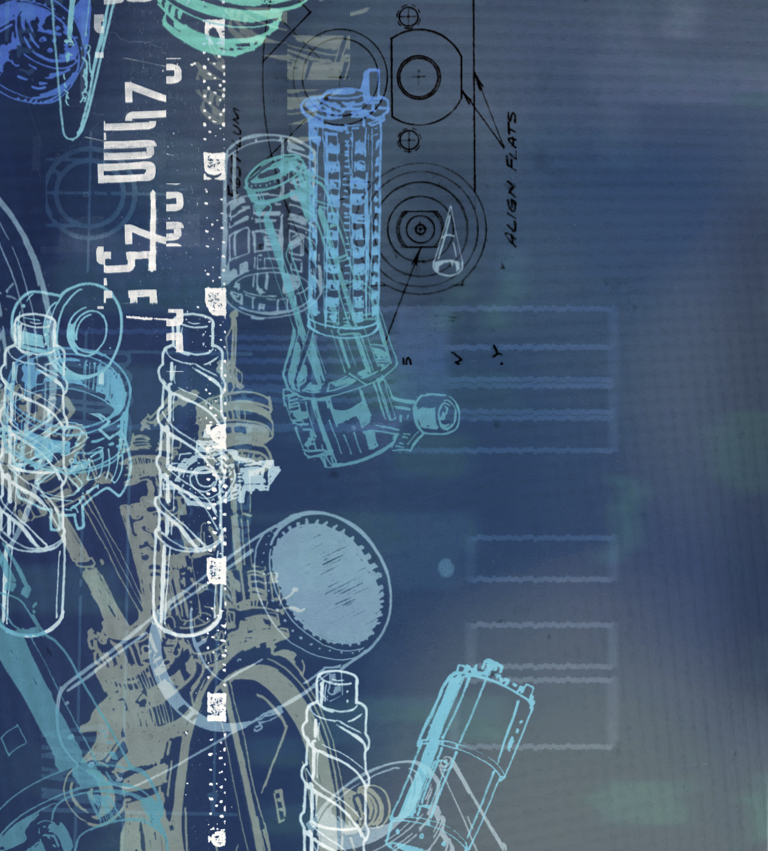
Over two years, the Nuclear Threat Initiative (NTI) and C4ADS worked together on a pilot project to demonstrate the viability of using PAI and machine learning to detect high-risk and/ or illicit nuclear trade.
Executive Summary #
For decades, illicit trade in nuclear materials, equipment, and technologies has undermined global nuclear non- proliferation efforts. Sophisticated actors establish front companies, forge documents, and launder money to obscure proliferation activities, and they too often are able to evade detection — even as they operate within legal systems of trade, finance, transportation, and communication.
They do leave footprints, however, and now, with an increase in the volume and variety of publicly available data, there are new opportunities to discover and expose such activities. When applied to the right forms of publicly available information (PAI), emerging data science methods and advanced analytical tools can expose proliferation activities, and they should be used to serve the global non-proliferation mission to reduce the risk of catastrophic consequences from use of a nuclear weapon.
Over two years, the Nuclear Threat Initiative (NTI) and the Center for Advanced Defense Studies (C4ADS) worked together on a pilot project to demonstrate the viability of using PAI and machine learning to detect high-risk and/ or illicit nuclear trade. The initiative leveraged NTI’s nuclear expertise and C4ADS’s data management, engineering, and analysis capabilities to identify high-risk nuclear proliferation activities at scale.
The project succeeded. Trade network analysis — and the machine learning processes that supported it — uncovered previously unknown entities of elevated risk within millions of transactions. The work showed that automated data preparation could save hundreds of analyst hours and help identify twice as many potentially high-risk entities as previous manual efforts. In addition, when applied to a baseline study of more than four million records, machine learning techniques could identify 50 new leads for further review. During the two-year study, at least ten entities identified through these approaches were added to a U.S. government export control list, demonstrating that novel analytic approaches to PAI can produce law enforcement-relevant insights.
Key Findings & Recommendations #
- The use of publicly available data and analysis is effective at identifying high-risk nuclear trade. To be operationally useful, the analysis approach must be able to accommodate the velocity, volume, variety, and complexity of real-world data.
- Machine learning tools can be used to dramatically enhance data analysis in terms of both speed and quality. Model selection is best accomplished empirically to determine the most effective models for particular situations. This method places a premium on access to relevant datasets as well as subject matter expertise.
- The use of PAI when coupled with machine learning tools holds enormous potential for the detection and prevention of illicit nuclear trade. Additional work is needed to address regional trade pattern differences, uneven data availability, and opportunities for integration with other data types.
The project recommends that leaders of non-proliferation efforts in governments and multi-lateral organizations around the world ensure that PAI and modern analytical approaches are employed to monitor and ultimately disrupt illicit nuclear activities. Specifically, our recommendations include the following:
- Integrating PAI more broadly into existing monitoring and verification regimes;
- Using modern analytical tools and approaches, including machine learning, to enable collection and analysis of PAI at scale;
- Building partnerships to allow analysts access to complementary data and capabilities; and
- Embracing the use of PAI and modern analytical tools for future international non-proliferation initiatives.
Taken together, these steps can support analytic production with the quality, scale, and timeliness required for an operational monitoring capability. Indeed, in the future, it may be impossible for a proliferator to evade detection.